Predicitions of Rock Burst Tendencies Based on Principal Component Analysis and GWO-SVM Model
-
摘要: 为解决深部地下工程岩爆倾向性预测问题,提出主成分(PCA)与改进支持向量机(GWO-SVM算法)组合的岩爆倾向性预测模型。依据岩爆形成机理,选取围岩最大切向应力σθ、岩石单轴抗压强度σc、岩石单轴抗拉强度σt、岩石应力系数σθ/σc、岩石脆性系数σc/σt、弹性能量指数Wet作为岩爆倾向性评价指标,利用PCA优化评价指标结构,获得3个符合岩爆特征的评价指标(F1、F2、F3),减小了计算的复杂度。搜集国内外64组岩爆实测案例构建原始岩爆数据集,将原始岩爆数据集与PCA预处理后的岩爆数据集分别作为4种机器学习模型(PNN模型、Elman模型、SVM模型、GWO-SVM模型)的输入向量,综合模型预测准确率、运行时间等方面,对比不同输入组合下各模型的预测性能。结果表明:经PCA优化后模型整体性能有了显著提升(准确率提升了6.25%~12.5%,运行时间缩短了11.20%~58.42%),且与PCA结合的GWO-SVM模型预测准确率最高可达93.75%。Abstract: To predict rock burst tendencies in deep underground engineering, a prediction model of rock burst tendencies combining the principal component analysis (PCA) and improved GWO-SVM algorithm was proposed. Based on the formation mechanism of rock burst, the maximum tangential stress of rock σθ, the uniaxial compressive strength of rock σc, the uniaxial tensile strength of rock σt, the rock stress coefficient σθ/σc, the rock brittleness coefficient σc/σt, and the elastic energy index Wet were selected as estimate indexes for rock burst tendencies, and the three estimate indexes including F1, F2 and F3 were obtained to optimize estimation index structure by PCA,which reduced the complexity of calculations. The original data set of rock burst was constructed by collecting 64 sets of rock burst cases at home and abroad, and the original rock burst data set and the pre-processed rock burst data set by PCA were used as input vectors of four machine learning models including the PNN model, the Elman model, the SVM model and the GWO-SVM model, and the prediction performances of each model were compared in different combinations of inputs in terms of prediction accuracy and running times. The results indicated that the overall performances of models were significantly improved after optimization by PCA. Comparison with those models before optimization by PCA, the calculation accuracy of improved models was enhanced by 6.25% to 12.5%, running times was shortened by 11.20% to 58.42%, and the prediction accuracy of the GWO-SVM model combined with PCA was up to 93.75%.
-
[1] 任燕娟,梁士奎.基于模糊物元理论的岩爆倾向性等级评价[J].中国矿业,2020,29(6):149-154,175. [2] 汤志立,王雪,徐千军.基于过采样和客观赋权法的岩爆预测[J].清华大学学报(自然科学版),2021,61(6):543-555. [3] 张恒源,范俊奇,郭佳奇,等. 基于多参量判据的深地下工程岩爆倾向性研究[J]. 高压物理学报,2022,36(2):176-189. [4] BROWN E T, HOEK E. Underground excavations in rock[M]. London:CRC Press,1980. [5] TAO Z Y. Rock burst and its discrimination in high ground stress zone [J]. Renmin Changjiang River, 1987(5):25-32. [6] 徐林生,王兰生.二郎山公路隧道岩爆发生规律与岩爆预测研究[J].岩土工程学报,1999(5):569-572. [7] 李晋,周宗红,丁文俊.基于主成分物元可拓模型的岩爆倾向性预测[J].化工矿物与加工,2022,51(5):7-12. [8] 黄明健,王加闯,过江.基于RS-改进云模型的岩爆倾向性预测[J].有色金属(矿山部分),2019,71(5):93-101. [9] 赵国彦,李振阳,梁伟章,等.岩爆预测的Vague集模型[J].矿冶工程,2018,38(1):1-4,10. [10] 刘冉,叶义成,张光权,等.岩爆分级预测的粗糙集-多维正态云模型[J].金属矿山,2019(3):48-55. [11] 赵亚东,杨帆,张庆,等.基于信念网络的岩爆倾向性预测模型研究[J].矿业安全与环保,2018,45(3):80-85. [12] 杨玲,魏静.基于支持向量机和增强学习算法的岩爆烈度等级预测[J].地球科学,2023,48(5):2011-2023. [13] 张馨月,宋绍成.突发事件中基于支持向量机算法的文本分类研究[J].信息技术与信息化,2022(8):13-16. [14] 李佳园,李耀南,惠继录.灰狼优化算法的应用综述[J].数字技术与应用,2022,40(9):10-13. [15] 赵珂劼,池恩安,赵明生,等.主成分分析法在露天煤矿岩石质量评价中的应用[J].爆破,2019,36(3):50-55. [16] 叶涛,韦阿娟,黄志,等.基于主成分分析法与Bayes判别法组合应用的火山岩岩性定量识别:以渤海海域中生界为例[J].吉林大学学报(地球科学版),2019,49(3):873-880. [17] MIRJALILI S, MIRJALILI S M, LEWIS A. Grey wolf optimizer[J]. Advances in Engineering Software,2014,69:46-61. [18] 宋玉生,刘光宇,朱凌,等.改进的灰狼优化算法在SVM参数优化中的应用[J].传感器与微系统,2022,41(9):151-155. [19] 杨悦增,邓红卫,虞松涛.基于随机森林模型的岩爆等级预测研究[J].矿冶工程,2017,37(4):23-27. [20] 陈鹏宇,余宏明,师华鹏. 基于权重反分析和标准化模糊综合评价的岩爆预测模型[J]. 岩石力学与工程学报,2014,33(10):2154-2160. -
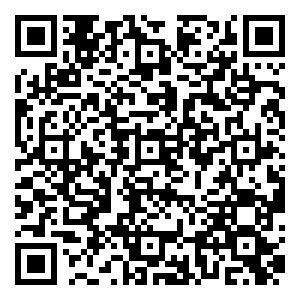
计量
- 文章访问数: 114
- HTML全文浏览量: 26
- PDF下载量: 1
- 被引次数: 0