LSTM-BASED DAMAGE PREDICTION AND ASSESSMENT OF SPATIAL FRAME STRUCTURE
-
摘要: 以凯威特型(K6)球面网壳为研究对象,研究基于数据驱动的空间网格结构的损伤预测和评估。通过数值模拟得到大气均匀腐蚀作用下结构模态频率的结构健康监测(SHM)模拟数据,基于长短期记忆(LSTM)神经网络,建立了结构损伤预测和评估的深度学习模型。最后,总结了基于LSTM神经网络的空间网格结构损伤预测和评估方法。结果表明:LSTM神经网络可以对SHM数据建立基于数据驱动的深度学习模型,对结构健康状态进行预测和评估。建立的模型在模拟数据上表现良好,具有良好的抗噪性,能很好地拟合SHM模拟数据趋势。利用更新后的数据集重新调整模型,可以达到持续对结构健康状态预测和评估的目的。Abstract: The Kiewitt (K6) spherical reticulated shell was used as the research object to study the data-driven damage prediction and assessment of the space frame structure. By numerical simulations, Structural Health Monitoring (SHM) simulation data of structural modal frequency subjected to uniform atmospheric corrosion were obtained. A Long-Short-Term Memory (LSTM) based the deep learning model for structural damage prediction and assessment was constructed. Finally, the LSTM-based damage prediction and assessment method for the space frame structure was summarized. The results showed that LSTM could be used to establish a data-driven deep learning model for SHM data, predict and assess the structural health status. The model performed well on the simulation data with good anti-noise properties which could nicely fit SHM simulation data with a good short-term prediction effect. The updated data sets could readjust the model, so as to achieve the continuous prediction and assessment for structural health status.
-
Key words:
- spatial frame structure /
- LSTM /
- damage prediction and assessment /
- deep learning
-
[1] 汪菁. 深圳市民中心屋顶网架结构健康监测系统及其关键技术研究[D]. 武汉:武汉理工大学, 2008. [2] 秦杰, 徐瑞龙, 徐亚柯, 等. 国家体育馆安全监测系统研究[J]. 施工技术, 2009, 38(3):40-43. [3] 李宏男, 杨礼东, 任亮, 等. 大连市体育馆结构健康监测系统的设计与研发[J]. 建筑结构学报, 2013, 34(11):40-49. [4] 罗尧治, 苑佳谦. 大跨度空间结构安全预警评估技术研究[J]. 空间结构, 2011, 17(3):61-68. [5] GOODFELLOW I, BENGIO Y, COURVILLE A. Deep Learning[M]. Canbridge:MIT Press, 2016:373. [6] GOODFELLOW I, BENGIO Y, COURVILLE A. 深度学习[M]. 赵申剑, 黎彧君, 李凯, 等, 译.北京:人民邮电出版社, 2017:319. [7] PASCANU R, MIKOLOV T, BENGIO Y. On the Difficulty of Training Recurrent Neural Networks[C]//International Conference on Machine Learning. 2013:1310-1318. [8] BENGIO Y, SIMARD P, FRASCONI P. Learning Long-Term Dependencies with Gradient Descent is Difficult[J]. IEEE Transactions on Neural Networks, 1994, 5(2):157-166. [9] HOCHREITER S, BENGIO Y, FRASCONI P, SCHMIDHUBER J. Gradient flow in recurrent nets:the difficulty of learning long-term dependencie[G]//KOLEN J F, KREMER S C.A Field Guide to Dynamical Recurrent Neural Networks. Piscataway:Wiley-IEEE Press, 2001:237-243. [10] HOCHREITER S, SCHMIDHUBER J. Long Short-Term Memory[J]. Neural Computation, 1997, 9(8):1735-1780. [11] 梁彩凤, 侯文泰. 碳钢、低合金钢16年大气暴露腐蚀研究[J]. 中国腐蚀与防护学报, 2005(1):2-7. [12] 中华人民共和国住房和城乡建设部. 空间网格结构技术规程:JGJ 7-2010[S]. 北京:中国建筑工业出版社, 2010. -
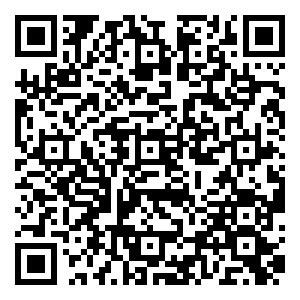
计量
- 文章访问数: 121
- HTML全文浏览量: 11
- PDF下载量: 4
- 被引次数: 0